A new promising phylogenetic marker to study the diversity of fungal communities: Predotar is designed to predict the presence of mitochondrial and plastid targeting peptides in plant sequences. It uses an SVM-based approach with a custom kernel that employs a composite spectrum or multiple k -mer encoding conjoined with a bit vector indicating the presence or absence of a range of sequence motifs known to be important for nuclear proteins. Molecular Biology of the Cell, 4th edn. Journal List Nucleic Acids Res v. Conflict of interest statement. LIPS Adamian and Liang, MemPype Pierleoni et al, is a pipeline for identifying membrane-associated proteins and discriminates types of membrane SCLs and topology for eurkaryotic membrane proteins.
Uploader: | Vudokree |
Date Added: | 6 July 2016 |
File Size: | 16.46 Mb |
Operating Systems: | Windows NT/2000/XP/2003/2003/7/8/10 MacOS 10/X |
Downloads: | 10039 |
Price: | Free* [*Free Regsitration Required] |

PSLDoc Chang et al, uses document classification techniques and incorporates a probabilistic latent semantic analysis with a support vector machine model, for prediction on prokaryotes and eukaryotes. UniProt ID, localization site, the distance in localization features from the query, the percent identity to the query, a link to its UniProt entry, the subcellular localization line from UniProt and other available localization information. Psotr Glycoside Hydrolase 63 wopf. LIPS Adamian and Liang, MemPype Pierleoni et al, is a pipeline for identifying membrane-associated proteins and discriminates types of membrane SCLs and topology for eurkaryotic membrane proteins.
WoLF PSORT: protein localization predictor.
Mimicking cellular sorting improves prediction of subcellular localization. It uses an SVM-based approach with a custom kernel that employs a composite spectrum or multiple k -mer encoding conjoined with a bit vector indicating the presence or absence of a range of sequence motifs known to be important for nuclear proteins. Paort Tsaousis et al, is a database of experimentally derived topological models of transmembrane proteins.
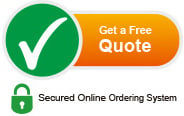
LOChom is a database of predicted localizations based on homology to experimentally annotated proteins. AMPDB Heazlewood and Millar, is woolf database of known and predicted mitochondrial proteins in the plant species Arabidopsis thaliana.
Bilipid membranes divide eukaryotic cells into various types of organelles containing characteristic proteins and performing specialized functions. Integrative proteome analysis of Brachypodium distachyon roots and leaves reveals a synergetic responsive network under H2O2 stress.
WoLF PSORT
Yu et al,v2. NESbase la Cour et al, is a database with a collection of nuclear export signals. Viral protein subcellular localization predictors: Active Virus-mPLoc Shen and Chou, predicts viral protein subcellular localization with the ability to predict multiple localizations for a protein.
The localization features for the query and its neighbors are shown.
By clicking accept or continuing to use the site, you agree to the terms outlined in our Privacy PolicyTerms of Serviceand Dataset License. Machine learning approaches for the prediction of signal peptides and other protein sorting signals. The page is currently hosted by the Brinkman Laboratory at Simon Fraser University, and our goal psor to provide an open-source resource centre for researchers interested in subcellular localization prediction.
ClarosPierre Vincens. BaCelLo Pierleoni et owlf, is a predictor for five classes of eukaryotic subcellular localization secretory pathway, cytoplasm, nucleus, mitochondrion and chloroplast and it is based on different SVMs organized in a decision tree. Signal Peptides Menne at al, Previous version was described in Heddad et al, paper. Subnuclear Wof Prediction System Lei and Dai,Psortt and Dai, predicts subnuclear localization by combining an SVM-based system for sequence analysis with a nearest-neighbor classifier using a similarity measure derived from the GO annotation terms for the protein sequences.
LocDB Rastogi and Rost, is a manually curated database with experimental annotations for the subcellular localizations of proteins in Homo sapiens and Arabidopsis thaliana. The different methods they benchmarked were found to have different strengths. SubLoc Hua and Sun, uses Support Vector Machine to assign a prokaryotic protein to the cytoplasmic, periplasmic, or extracellular sites, and a eukaryotic protein pssort the cytoplasmic, mitochondrial, nuclear, or extracellular sites.
WoLF PSORT: protein localization predictor.
SecretP Yu et al, predicts mammalian secreted proteins using PseAA and SVMs SherLoc2 Briesemeister et al, predicts animal, plant and fungal protein subcellualr localizations using sequence-based and text-based features.
Jennifer Gardy and Fiona Brinkman: For large-scale use, owlf as whole genome annotation, we encourage users to download the stand-alone package available on the server and run WoLF PSORT locally. At the bottom of the page, we have also provided a suggested reading list containing selected review articles describing SCL and SCL prediction. For convenience, sequence alignments of the query to similar proteins and links to UniProt and Gene Ontology are provided.
Identification of prokaryotic and eukaryotic signal peptides and prediction of their cleavage sites. LOCtree3 Goldberg et al, FFT-based SCL predictor Wang et al, is a wol Fourier transform-based support vector machine for subcellular localization prediction using different substitution models GNBSL Guo et al, generates subcellular localization prediction for Gram negative bacteria using a psoort of several different SVM's based on the PSSM and PSFM generated from the input protein HensBC Bulashevska and Eils, predicts localizations by constructing a hierarchical dolf of classifiers, namely Bayesian classifiers based on Markov chain models Wang et al, predict protein SCL by pseudo amino acid composition with a segment-weighted and features-combined approach.
No comments:
Post a Comment